In the rapidly evolving technological landscape, the debate surrounding the economic viability of AI (Artificial Intelligence) startups as opposed to SaaS (Software as a Service) companies is intensifying. Recent insights from industry stalwarts like Martin Casado and Matt Bornstein have further fueled this discourse, casting a spotlight on the fundamental business performance of AI-centric ventures.
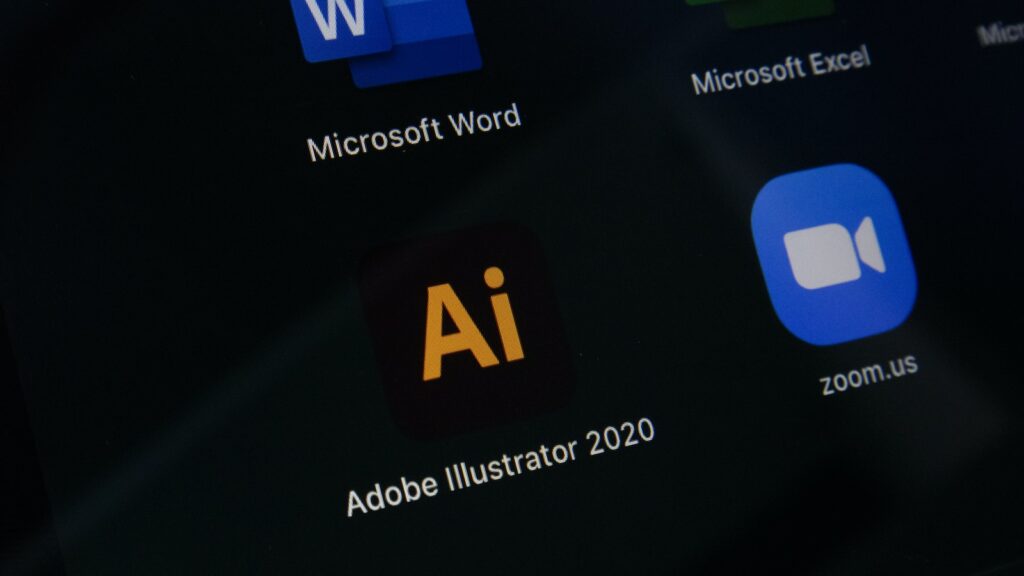
The AI vs. SaaS Controversy
At the heart of this debate lies a prevalent assumption: a significant portion of the industry expects AI enterprises to mirror the economic structures of traditional software companies. This belief, however, isn’t unanimous. Several industry experts voice concerns, challenging this widely accepted notion.
The reservations about AI startups primarily arise from their economic performance. Given the substantial attention and funding AI startups have attracted, any indication of them exhibiting less-than-expected favorable economics necessitates a thorough examination.
The Gross Margin Conundrum
One of the most debated aspects is the gross margin associated with AI-driven firms. A dominant school of thought posits that AI companies, due to their inherent operational nature, might work with narrower gross margins than SaaS entities. Several factors contribute to this perspective:
Cloud Computing Costs: AI operations, especially those involving deep learning models, require extensive computational power. This often translates to higher expenses on cloud services.
Human Intervention: Unlike traditional software, AI models require continuous training and fine-tuning, necessitating human expertise and thereby incurring additional costs.
Edge Cases and Differentiation: AI solutions, by their very nature, encounter numerous edge cases that need bespoke solutions. Moreover, the AI market is burgeoning, and many solutions overlap, making genuine product differentiation a challenge.
If this viewpoint holds water, the implications for AI startups are significant. It suggests that for every dollar of revenue they generate, their valuation might be less than that of a SaaS company. In essence, when it comes to revenue multiples, AI startups could be at a disadvantage.
The Capital Dynamics in AI
The discussion about the economics of AI startups becomes even more pertinent when one considers the vast amounts of capital these ventures have attracted. But is the skepticism about AI gross margins a universally accepted truth?
Engaging with seasoned investors, known for their AI-centric portfolios, offers a more nuanced understanding. Their insights paint a picture of diversity, potential, but also challenges in the AI startup arena.
Investor Insights on AI Economics
When probed about the economic dynamics of AI startups, three core areas emerged:
Margins: The consensus is that while AI startups might face challenges initially, as their solutions mature, they could potentially achieve margins comparable to SaaS companies.
Valuations: The initial margin dynamics might influence valuations, but the long-term potential of AI solutions, especially in sectors like healthcare, finance, and autonomous mobility, could drive higher valuations.
Optimism: Despite the challenges, there’s a palpable optimism about AI-driven ventures. The transformative potential of AI is undeniable, and while the journey might be riddled with hurdles, the destination promises significant rewards.
Diving Deeper with Examples and Case Studies
To truly understand the dynamics, let’s explore some examples, each supplemented with two case studies:
AI in Retail:
- Example: Personalized recommendation systems might have high setup costs, but the surge in sales can justify the investment.
- Case Study 1: FashionFits, an online clothing retailer, implemented an AI-driven recommendation system. Initial setup costs were substantial, but within six months, they reported a 30% increase in sales from repeat customers due to personalized shopping experiences.
- Case Study 2: TechGadgetry, an electronics e-commerce platform, integrated AI to suggest products based on browsing history. They witnessed a 20% uptick in cross-sales within three months.
SaaS in HR:
- Example: Tools for talent management might have predictable margins but face challenges in terms of user adaptability.
- Case Study 1: HireRight, an HR SaaS platform, offered advanced talent management tools. While their margins remained stable, they had to invest significantly in training modules due to the complexity of their software.
- Case Study 2: TalentFlow introduced an AI-driven candidate matching system. Despite its efficiency, they faced challenges in user adoption and had to roll out multiple tutorial sessions.
AI in Manufacturing:
- Example: Predictive maintenance solutions might require significant initial investment but can lead to substantial savings in the long run.
- Case Study 1: AutoMakers Inc., a car manufacturing company, integrated AI for predictive maintenance of their assembly lines. This reduced their machine downtimes by 40% annually.
- Case Study 2: SteelCraft, a metalworks factory, employed AI to predict wear and tear of their machinery. They reported savings of over $2 million in the first year by avoiding unplanned maintenance.
SaaS in Marketing:
- Example: Platforms offering campaign management tools could face margin pressures due to the plethora of similar tools available.
- Case Study 1: MarketMaven, a marketing SaaS, offered a unique campaign management tool. However, with many competitors offering similar features, they had to reduce their subscription prices.
- Case Study 2: PromoPro introduced a tool with AI-driven insights for campaign optimization. Despite its advanced features, they faced challenges in market differentiation.
AI in Real Estate:
- Example: Virtual tour assistants can enhance property viewing experiences, and while initial setup costs are high, they can lead to quicker property turnovers.
- Case Study 1: RealtyVisions introduced AI-driven virtual tour assistants for their property listings. This resulted in a 25% increase in property turnovers in the first quarter.
- Case Study 2: HomeScape Realtors employed AI to offer 3D virtual home tours. They reported a 50% increase in inquiries and a 20% increase in sales within six months.
SaaS in Collaboration:
- Example: Tools facilitating remote work have seen a surge, but with many players in the field, differentiation becomes a challenge.
- Case Study 1: CollabSpace, a remote work SaaS platform, saw a surge in subscriptions during the pandemic. However, with the emergence of multiple similar platforms, they faced challenges in retaining customers.
- Case Study 2: TeamSync introduced unique collaboration features, but with giants like Zoom and Microsoft Teams dominating the market, they had to invest heavily in marketing to carve a niche.
Concluding Thoughts
The juxtaposition of AI startups and SaaS companies offers a fascinating study in economics, potential, and challenges. While AI ventures might grapple with issues in their nascent stages, their potential to revolutionize industries is unparalleled. It’s imperative to view this debate from a holistic lens, understanding that the journey of each startup, be it AI or SaaS, is distinct and laden with its unique set of challenges and opportunities.